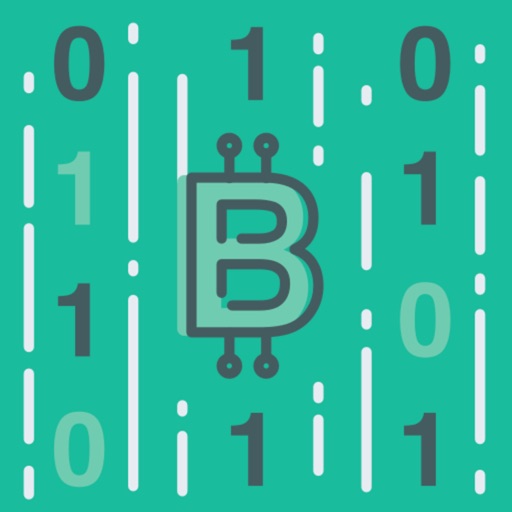
Stock market prediction is usually considered as one of the most challenging issues among time series predictions due to its noise and volatile features, moreover, if we're talking about cryptocurrency markets

Bitcoin Prediction
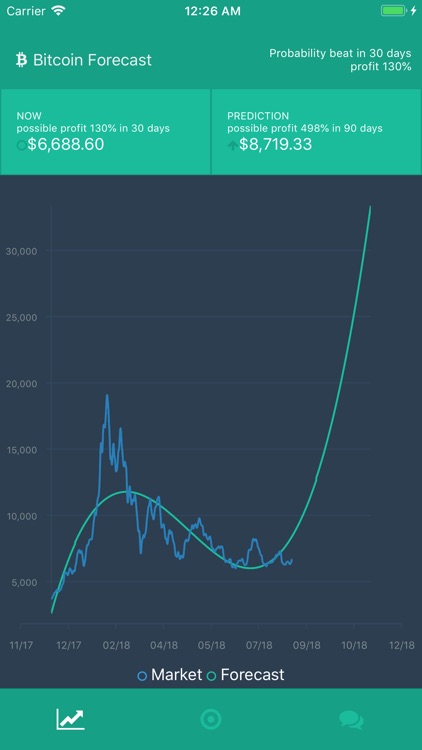
What is it about?
Stock market prediction is usually considered as one of the most challenging issues among time series predictions due to its noise and volatile features, moreover, if we're talking about cryptocurrency markets.
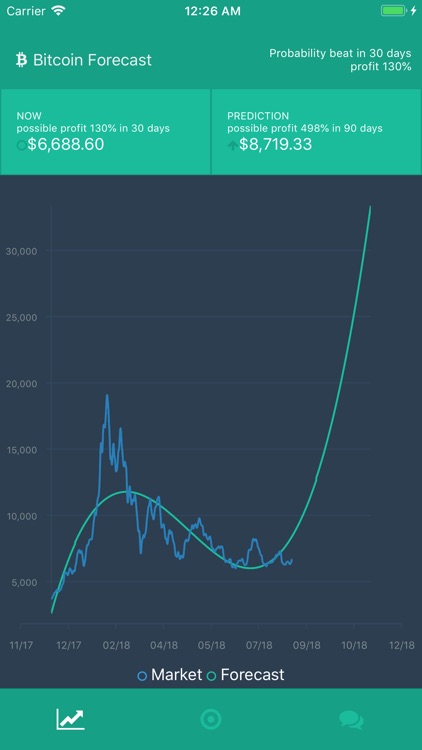
App Store Description
Stock market prediction is usually considered as one of the most challenging issues among time series predictions due to its noise and volatile features, moreover, if we're talking about cryptocurrency markets.
In this application, I will experiment with machine learning and bitcoin predictions.
To generate the deep and invariant features for step-ahead crypto stock value prediction, this work presents a deep learning framework for financial time series using a deep learning-based forecasting scheme that integrates the architecture of stacked autoencoders and long-short-term memory.
Long short-term memory is one of the many variations of recurrent neural network (RNN) architecture. In this section, the model of RNN and its LSTM architecture for forecasting the closing price is introduced. Initially, we started with the basic recurrent neural network model and then proceed to the LSTM model.
The RNN is a variety of deep neural network construction that has a deep structure in the transient dimension. The premise of a common neural network is that all units of the input vectors are free of each other. As a result, the traditional neural network cannot make use of the sequential information. In opposition, the RNN model combines a hidden state that is generated by the sequential data of a time series, with the output dependent on the hidden state.
The primary design of LSTM network holds the number of hidden layers with a number of stops, which becomes a number of past data that account for training and probing. The financial time series is separated into three subsets: a training subset, a validation subset, and testing subset, with a proportion of 50%, 30%, and 20% respectively. The back-propagation algorithm is applied to train the WSAEs-LSTM model as well as the models in the experimental control group including WLSTM, LSTM, and RNN. The speed of convergence is controlled by the learning rate, which is a decreasing function of time. The experimental result becomes solid once convergence is achieved through the combinations of parameters.
Future Development
At this moment I'm experimenting with the real-time market changes:
1. Orders
2. Market cap
3. 24h change
The application represents a tiny client for a neural-network that operating under GTX 1060 GPU. We're stuck a little limit RAM using 1060
More about network and models that developer implement, more or less described at my website.
DISCLAIMER: Bitcoin Forecast running experimental models, it may show you a profit or investment potential, BUT THE RISKS MUCH HIGHER. Until a neural network running under low GTX GPU, it's hard to guarantee accuracy. Any use for commercial purposes at your own risk.
AppAdvice does not own this application and only provides images and links contained in the iTunes Search API, to help our users find the best apps to download. If you are the developer of this app and would like your information removed, please send a request to takedown@appadvice.com and your information will be removed.